The Potential and Limitations of AI Emotion Analysis in Human Speech
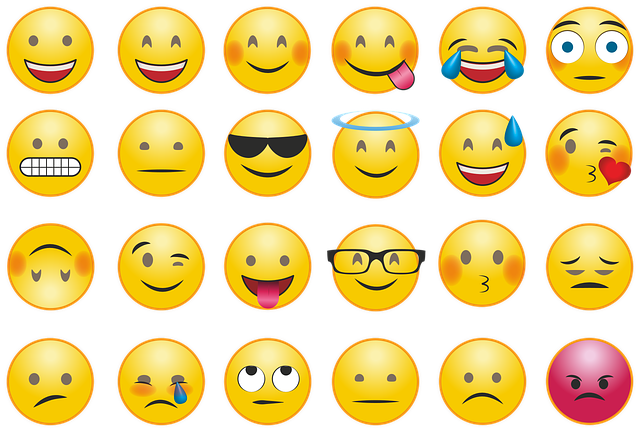
Introduction: Can AI Decode Human Emotions Through Voice?
Human speech is complex, rich with subtle cues that convey not just words but emotions, intent, and cultural context. While humans often intuitively pick up on these cues, the question arises: how well can artificial intelligence (AI) analyze emotions through voice? AI-powered emotion recognition has become a burgeoning field, promising applications in customer service, healthcare, education, and even personal relationships.
However, the reality is more nuanced. Cultural differences, linguistic variations, and the inherent complexity of emotions pose significant challenges. For example, Japanese speech is often calm and subdued, while Shanghainese might sound argumentative to outsiders despite being perfectly neutral in meaning. Furthermore, critics argue that even if AI identifies emotions like "positive" or "negative," this information alone is often not actionable, much like sentiment analysis in social media.
This article explores how AI attempts to analyze human emotions through voice, the challenges it faces, and whether this technology is truly useful or just a technical curiosity.
1. How AI Analyzes Emotions in Voice
AI systems analyze emotions in speech by processing vocal features such as tone, pitch, volume, and rhythm. Here’s how it works:
1.1 Key Components of AI Emotion Analysis
-
Acoustic Features:
- Pitch: High pitch might indicate excitement or anger, while low pitch often suggests calmness or sadness.
- Volume: Louder speech can reflect anger or enthusiasm, whereas softer tones might indicate fear or sadness.
- Rhythm and Pauses: Rapid speech might signal urgency, while long pauses can indicate hesitation or thoughtfulness.
-
Machine Learning Models:
- AI models are trained on large datasets of labeled speech to identify patterns corresponding to specific emotions.
-
Emotion Labels:
- Common emotion categories include happiness, sadness, anger, fear, and neutral. Advanced models may include more nuanced states like frustration or sarcasm.
-
Natural Language Processing (NLP):
- Some systems combine acoustic analysis with the meaning of words to refine emotion detection.
1.2 Current Capabilities of AI Emotion Analysis
AI emotion analysis is surprisingly effective in controlled environments, achieving accuracy rates of 70–90% in identifying basic emotions. This makes it suitable for applications like:
- Customer Service: Identifying frustrated customers during phone calls.
- Mental Health Monitoring: Detecting signs of depression or anxiety through voice patterns.
- Education: Gauging student engagement or confusion in online learning environments.
2. Challenges in Analyzing Emotions Through Voice
While promising, AI emotion analysis is far from perfect. Several challenges undermine its reliability and applicability in real-world scenarios.
2.1 Cultural Differences in Speech Patterns
Emotional expression varies significantly across cultures, making it difficult for AI to generalize.
- Japanese Speech: Known for its calm and polite tone, even in emotionally charged situations. This makes anger or frustration harder to detect.
- Shanghainese Speech: Its naturally loud and emphatic tone might be misinterpreted by AI as anger when it’s just a cultural norm.
- Western Speech: In English-speaking countries, emotions are often expressed more openly, which can make analysis easier.
Without accounting for cultural context, AI risks misclassifying emotions, leading to inaccurate or even offensive conclusions.
2.2 Linguistic Variations
Even within a single language, accents, dialects, and individual speaking styles create variability.
- Example: A regional accent in English might emphasize certain sounds that AI misinterprets as emotional cues.
2.3 The Complexity of Human Emotions
Emotions are rarely clear-cut. People often experience mixed emotions, like being happy and nervous simultaneously. AI struggles to detect such subtleties.
- Example: Sarcasm is particularly challenging for AI, as it relies on tone and context that are hard to quantify.
2.4 Ambient Noise and Real-World Conditions
Background noise, poor audio quality, and interruptions can distort speech signals, reducing the accuracy of AI analysis.
- Example: In a noisy customer service call, AI might interpret a customer’s raised voice as anger when they’re merely trying to be heard.
3. The "Actionability" Debate: Is Emotion Detection Useful?
Critics argue that identifying emotions like "positive" or "negative" is often not actionable. Simply knowing someone is frustrated doesn’t automatically reveal how to address the issue.
3.1 The Social Media Parallel
In social media sentiment analysis, AI often labels posts as positive, neutral, or negative. While useful for broad trends, these labels don’t offer actionable insights.
- Example: A "negative" tweet about a product might reflect a minor complaint or a significant defect. Without deeper context, the sentiment score has limited value.
3.2 The Same Problem in Voice Analysis
Similarly, in voice emotion analysis:
- Customer Service: Knowing a caller is angry doesn’t specify whether they’re upset about billing, product quality, or something else.
- Healthcare: Detecting sadness in a patient’s voice might indicate depression—or simply a bad day.
3.3 Bridging the Gap to Actionable Insights
To be actionable, emotion detection needs to be paired with:
- Contextual Understanding: Combining vocal analysis with the actual content of speech.
- Personalization: Recognizing individual differences in emotional expression.
- Automated Responses: Suggesting specific actions, like escalating a call to a supervisor or offering personalized resources.
4. Potential Applications of Emotion Analysis
Despite its challenges, AI emotion analysis has exciting potential in various fields:
4.1 Customer Support
- Proactive Assistance: Automatically escalating calls with angry customers to experienced agents.
- Training: Providing feedback to agents on how their tone impacts customer satisfaction.
4.2 Healthcare
- Mental Health Monitoring: Identifying early signs of depression or anxiety in patients.
- Telemedicine: Enhancing virtual consultations by analyzing patient tone alongside verbal descriptions.
4.3 Education
- Student Engagement: Tracking whether students are confused or bored during online classes.
- Personalized Feedback: Adapting teaching styles based on emotional responses.
4.4 Law Enforcement
- Crisis Intervention: Detecting stress or fear in emergency calls to prioritize urgent cases.
- Interrogations: Analyzing suspect emotions to guide questioning strategies.
5. Can AI Improve Over Time?
Advancements in AI and machine learning hold promise for overcoming the current limitations of emotion analysis. Key areas of development include:
5.1 Multimodal Analysis
Combining voice with facial expressions, body language, and physiological signals (e.g., heart rate) could improve accuracy.
- Example: Detecting both a trembling voice and a flushed face might confirm nervousness.
5.2 Cultural Sensitivity Training
AI models can be trained on diverse datasets to account for cultural and linguistic variations.
- Example: Including Shanghainese speech patterns in training data to distinguish natural tone from anger.
5.3 Real-Time Adaptation
Future AI systems could learn and adapt to individual communication styles during interactions, improving personalization.
- Example: Recognizing that a specific customer tends to speak loudly even when calm.
6. A Balanced Perspective: The Human Touch Matters
While AI emotion analysis offers exciting possibilities, it is unlikely to fully replace human intuition and empathy. Instead, it should complement human efforts:
6.1 Augmenting Human Abilities
- AI can handle repetitive tasks and provide initial insights, freeing humans to focus on complex, high-value interactions.
6.2 Ethical Considerations
- Businesses must ensure that emotion analysis is used responsibly, respecting privacy and avoiding misuse.
7. Conclusion: The Promise and Pitfalls of AI Emotion Analysis
AI’s ability to analyze human emotions through voice is an exciting technological frontier. It holds the potential to transform industries like customer service, healthcare, and education. However, its effectiveness is limited by cultural differences, linguistic nuances, and the inherent complexity of human emotions.
To truly make emotion detection actionable, AI systems must evolve to incorporate context, personalization, and multi-modal analysis. At the same time, we must recognize the irreplaceable value of human intuition and empathy in understanding and addressing emotions.
As AI continues to develop, its role will likely shift from attempting to "replace" human understanding to enhancing and supporting it—creating a future where technology and humanity work hand in hand.
Examples of Communicative Challenges Across Languages
- Japanese: Subdued tone and limited emotional expression make it difficult for AI to detect strong emotions like anger or joy.
- Shanghainese: Emphatic tone can mislead AI into detecting conflict when the conversation is neutral.
- Italian: Expressive gestures and dramatic intonation might exaggerate emotions, confusing AI models.
- English: Variability in accents (e.g., Southern U.S. vs. British English) complicates the interpretation of tone.
Understanding these nuances is critical to developing AI that accurately analyzes and responds to human emotions.